Digital Framework: Automation and Augmentation
- John Vassh
- Feb 28
- 5 min read
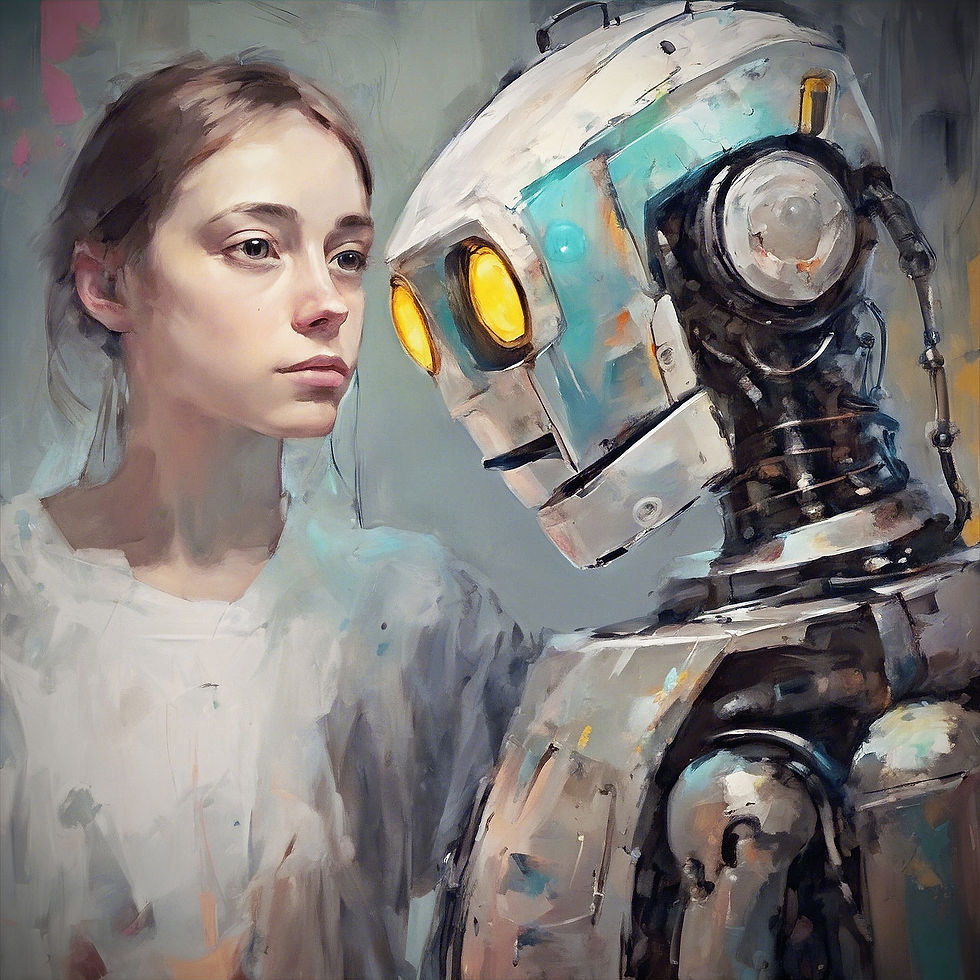
The landscape of artificial intelligence offers diverse capabilities, broadly distinguishable as either automative or augmentative. Automative AI is fundamentally about replacement; its core objective is to replicate and supplant human effort within a given task. The driving force behind this approach is to achieve heightened efficiency and speed, performing tasks at scales and paces unattainable by humans. Consistency and reliability are also paramount, aiming to minimize human error and ensure predictable, repeatable outcomes. Economically, automative AI offers the promise of cost reduction through optimized resource utilization and decreased labor expenses. Its inherent scalability makes it well-suited for handling vast volumes of work, particularly in domains characterized by repetitive, rule-based activities, which are often considered monotonous or tedious for human workers.
Current examples of automative AI abound, from systems that automatically extract data from forms and populate databases, eliminating manual data entry, to customer service chatbots designed to resolve simple inquiries without human agent intervention. Robotic process automation exemplifies this, with software robots mimicking human actions to automate workflows across multiple platforms and applications. In manufacturing, automated quality control using advanced vision systems replaces manual inspection, and the ongoing development of self-driving vehicles aims to automate your morning and evening commute.
When considering the implementation of automative AI, several factors deserve careful attention. The task itself must be meticulously evaluated: is it truly rule-based and well-defined, lending itself to algorithmic execution? Is it genuinely repetitive and mundane for human workers, such that automation would free them for more engaging endeavors? Crucially, is complete or near-complete automation even feasible and desirable for this task? Data is another critical element. Automative AI typically demands large quantities of high-quality training data to learn effectively and perform reliably. The data must also be representative of the real-world scenarios the AI will encounter to avoid skewed or ineffective performance.
Risk and error handling are paramount; the potential ramifications of errors made by an automated system must be thoroughly assessed, especially in critical domains. Robust error detection, fail-safes, and contingency plans for handling exceptions or system failures are essential, often necessitating some level of human oversight or intervention. Biases embedded within the AI itself can lead to unfair or discriminatory outcomes if not carefully designed and monitored, and transparency in the AI's decision-making processes is crucial for building trust and accountability, particularly in sensitive applications. Finally, the practical aspects of implementation, such as the upfront investment, the complexity of deployment, and the ongoing maintenance requirements, along with the need for internal expertise or external vendor support, must be weighed carefully.
In contrast to automative approaches, augmentative AI is centered on enhancement rather than replacement. Its fundamental purpose is to amplify and extend human capabilities, acting as a powerful tool or assistant to human experts. Here, human expertise, judgment, and control remain at the core, with AI serving to elevate human performance. Augmentative AI aims to improve decision-making by providing insightful analysis, predictions, and data-driven support to inform human judgment. It seeks to increase human productivity by making tasks faster, more efficient, and less error prone.
Furthermore, it fosters creativity and innovation by generating novel ideas, options, and perspectives for humans to explore and refine. Personalization and customization are also key benefits, enabling tailored experiences and information delivery to individual human needs. This approach is particularly well-suited for complex, nuanced tasks requiring human intuition, empathy, and sophisticated judgment.
Examples of augmentative AI are diverse and impactful. AI-powered diagnostic tools assist doctors by analyzing medical images and highlighting anomalies, while the physician retains the final diagnosis. AI writing assistants aid writers with grammar, style, and idea generation, while the writer maintains creative control and authorship. AI-driven CRM systems analyze customer data to provide insights to sales and marketing teams, empowering personalized interactions managed by human agents. Code completion and debugging tools augment programmers' abilities, and AI-enhanced accessibility tools provide real-time captions and image descriptions for people with disabilities, increasing information accessibility. And for our soldiers on the front lines, soon augmented reality headsets will encapsulate all critical variables on the battlefield for advanced networking and decision-making capabilities.
The successful deployment of augmentative AI hinges on the consideration of several factors, but primarily the human and machine collaboration. The interface and workflow must be designed for intuitive interaction, supporting effective partnership between humans and the artificial system. The level of human skill required to effectively utilize the tool is crucial, demanding appropriate training and a user-centric design. Building trust between humans and AI is paramount; users must understand the capabilities and limitations to confidently rely on and trust its suggestions.
The focus must consistently remain on human empowerment, ensuring the AI acts as a partner, not a substitute. Augmentative AI should amplify human strengths, targeting tasks where it can genuinely enhance human expertise. Explain ability and transparency are especially vital in augmentative systems, particularly in critical domains. Users often need to understand the AI's reasoning behind its suggestions, and the system should ideally allow for human override or adjustment of AI-generated recommendations. Continuous learning and improvement are inherent advantages of augmentative AI; systems should ideally learn from human feedback and adapt to evolving user needs and requirements, with ongoing evaluation and refinement processes in place.
Quick Reference Distinction
Feature | Automative AI | Augmentative AI |
Core Goal | Replacement of Human Effort | Enhancement of Human Capability |
Human Role | Replaced or Minimized | Central, Enhanced, Empowered |
Task Type | Repetitive, Rule-Based, High Volume | Complex, Judgement-Based, Creative, Nuanced |
Focus | Efficiency, Speed, Cost Reduction, Scale | Effectiveness, Quality, Innovation, Personalization |
Outcome | Independent Operation, Automated Process | Human-AI Collaboration, Enhanced Human Output |
Decision-Making | AI-Driven, Algorithmic | Human-Led, AI-Supported |
Error Tolerance | Low (often needs high reliability) | Can be higher (human oversight & correction) |
Data Needs | Often Large, High Quality, Representative | Can vary, but still data-driven, Small amounts of really good data > Large amounts of garbage data |
Essentially, choosing between automative and augmentative AI isn't a simple yes-or-no question. It's a strategic decision that depends on the task, what you want to achieve, balancing efficiency with boosting human abilities, ethical considerations, whether it's technically possible, and if people will actually use it. You don't have to be a software engineer or programmer to care about this; if you use modern software, you're already interacting with it, since most products now let you automate or augment tasks within them.
During a recent interview, Anduril founder Palmer Luckey, a leading voice in AI development, highlighted the critical distinction between automation and augmentation. He warned against the dangers of granting agentic AI autonomous control over nuclear weapons, a scenario eerily similar to Skynet's apocalyptic actions in the "Terminator" films. This prospect should be deeply unsettling and demands a rejection of such unmitigated automation. Hasta la vista, baby, to that approach.
Often, the most effective strategies involve a harmonious blend of both approaches, automating routine tasks to free human intellect for more strategic and creative endeavors, which are then further amplified by augmentative AI tools. The key lies in a thorough analysis of needs, goals, and context to determine the most appropriate and ethical way to harness the beast. Thank you for the privilege of your time.
Learn More: Anthropic, a leader in shaping AI development, shares findings on current use, implications, and economic impact.
